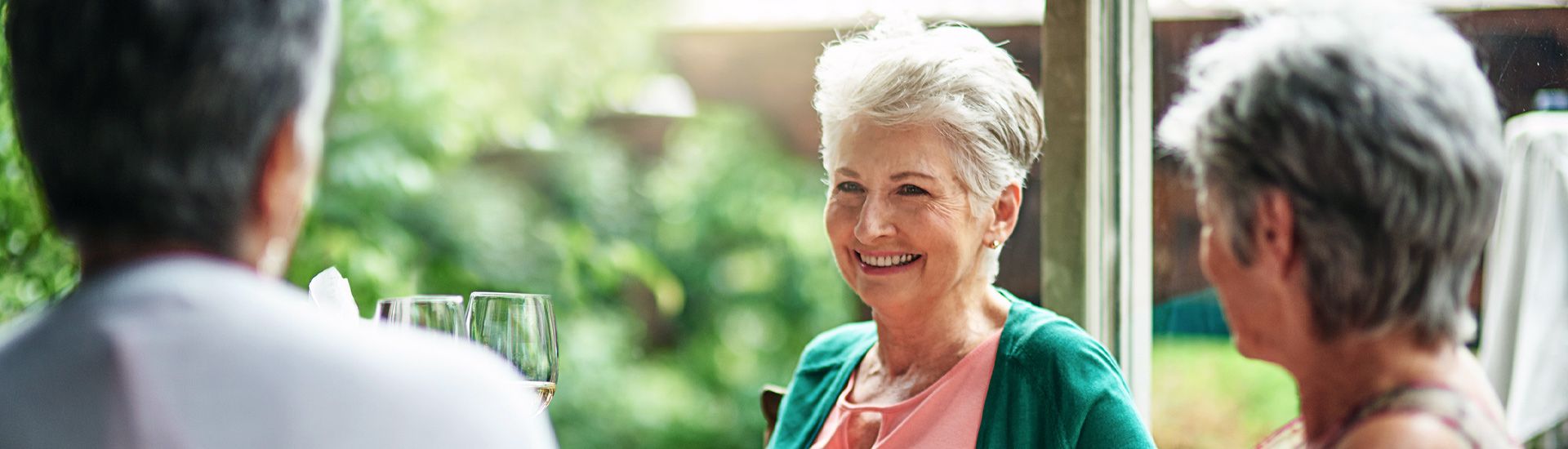
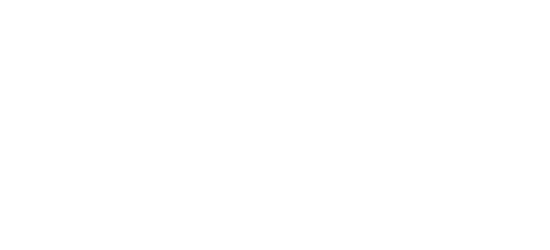
The Administration for Community Living (ACL), in collaboration with the Centers for Medicare & Medicaid Services (CMS), have launched a project to explore the use of predictive analytics as one component of a broader strategy to predict and prevent adult and elder maltreatment. The project, Predicting Risk of Adult Maltreatment, or PRAM will leverage artificial intelligence, machine learning, and other “big data” tools to investigate patterns of risk and protective factors across multiple data sources to determine if there is an association with reported incidence of adult maltreatment. The goal of the project is to create and improve interventions to prevent, and effectively intervene in, adult and elder maltreatment, and as an outcome, improve disabled and older adults’ quality of life and health quality outcomes, and reduce health care expenses.
While used extensively in other fields, predictive analytics is a relatively new concept for home- and community-based services and for understanding interpersonal violence, particularly adult and elder maltreatment. Predictive analytics generally refers to the use of a range of statistical techniques such as predictive modeling and data mining that use current and historical data to make predictions about future events and uses powerful and flexible technology tools. In the human services field, child welfare programs have been early adopters of predictive analytic approaches. While still relatively new, several states and counties have begun to invest in predictive analytic approaches to help prevent child abuse and neglect, particularly deaths and serious injury, as well as to avoid other negative outcomes associated with long-term involvement in the child welfare system.
Initiatives by other federal agencies have presented new opportunities for CMS and ACL to move forward in this area. This work necessitates close collaboration among social science subject matter experts; data scientists; and cloud computing architects, developers, and integrators to develop and test algorithms that may allow risk factors to be identified and applied to data to determine the probability of abuse, exploitation, and maltreatment of older and disabled adults at the community and individual levels.
Phase 1 launched in September 2019 with the purpose of understanding the following:
Phase 1 work included extensive literature reviews and environmental scans, as well as the convening of a technical expert panel to provide subject matter expert input to ACL on this project. During Phase 1, researchers and data scientists partnered to leverage Adult Protective Services (APS) data, via ACL’s National Adult Maltreatment Reporting System (NAMRS), and related risk factor data to predict APS system involvement at the county level for a subset of states. NAMRS data were associated with additional, publicly available data sources to develop algorithms that identified community- level risk factors associated with increased risk of APS system involvement. The results of this work demonstrated promise for this methodology, as well as elucidated some similarities and differences among the risk factors associated with different maltreatment experiences (e.g., any experience of maltreatment, self-neglect, maltreatment of individuals with adults with disabilities, and maltreatment of older individuals).
The second phase of the project, began September 2020. Phase 2 builds on the work of Phase 1, and will further explore the development of predictive analytic tools and algorithms that could be used as part of a protocol to identify risk factors and potential abuse of older and disabled adults first at the community-level, and then, after validation, at the individual-level. The experiment will use existing data sources, where possible, including NAMRS, Census data, and Medicaid Home and Community Based Services and Medicare payment data. A component of the experiment will be to assess the possibilities for associating disparate data sets to infer variables such as social isolation, cognitive function, dementia, and other identified risk factors.
Last Modified: 11/30/2023